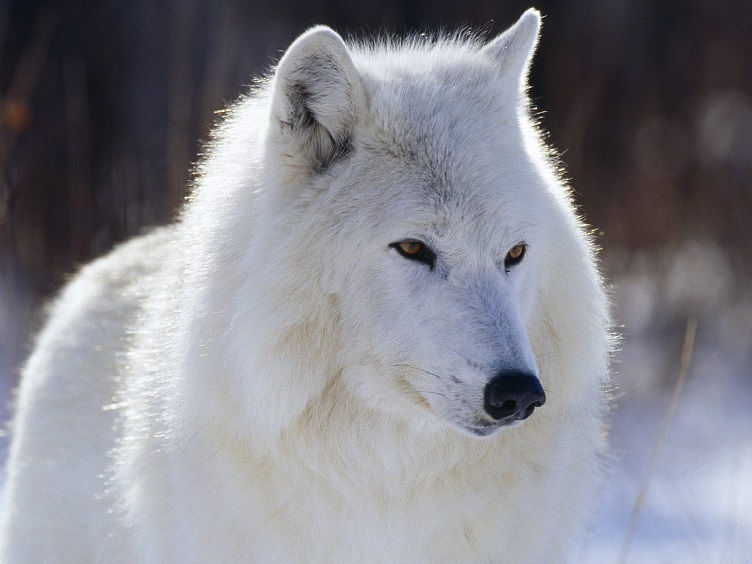
about me
Interests:
-
Software engineering
-
Data Science
-
Machine Learning
-
business analytics
​
I am currently pursuing a degree in Computer engineering from the university of Virginia. my goal is to become an engineer of Broad technical and interpersonal expertise. i am a dedicated engineer with a strong passion for learning both in and out of the classroom. outside of engineering, my other interests include ultimate DISC, running, and riffing on the guitar. At the Moment, I am exploring full-time opportunities after graduating in may of 2020.
Professional
Experience
Summer 2019
Fannie Mae
Software Intern
I worked on multiple different projects at this exciting financial company: loan data analysis/wrangling with Python, coding macros with VBA, and worked to build a geolocation app with Appian.
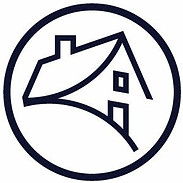
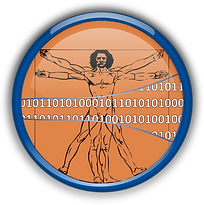
2017-present
INERTIA Lab
Data Analysis/ IoT Development Intern
I work as an undergraduate research assistant at UVA in the field of Smart and Connected Health. Specifically, I work on the Behavioral and Environmental Sensing and Intervention (BESI) smart home system to help caregivers take care of Dementia and cancer patients.
2016-2017
Dupont - Teijin Films
General Engineering Intern
At a film manufacturing plant in Hopewell, VA, I completed tasks in Document Control, profile management of manufacturing machinery, and the schematic design of power control systems.

2016
Penn Station East Coast Subs
Cashier
At my first job, I learned valuable customer service skills through working in a rigorous restaurant environment.
Projects
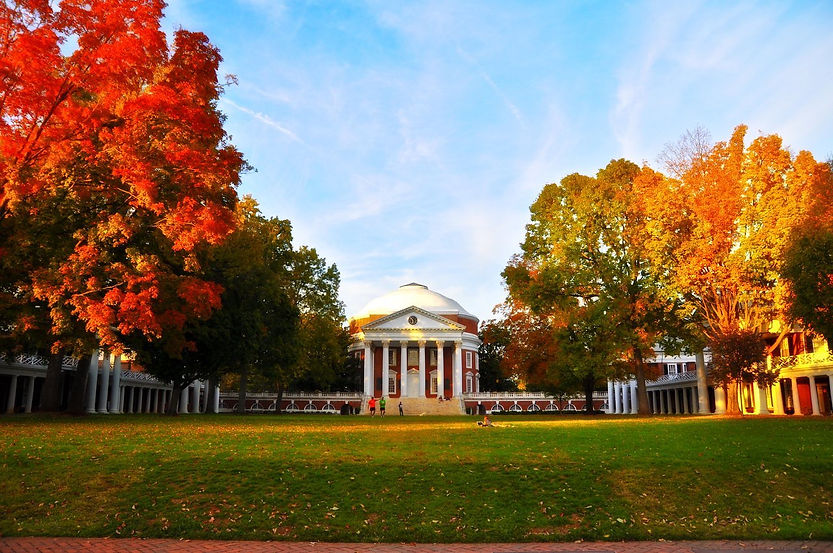